Inferencia causal en investigación educativa: Análisis de la causalidad en estudios observacionales de carácter transversal
DOI:
https://doi.org/10.30827/relieve.v29i2.26843Palabras clave:
Análisis causal, metodología estadística, evaluación, análisis de datosResumen
La suposición de relaciones causa-efecto en la investigación ex post facto es un problema ampliamente conocido en el ámbito de la metodología de investigación en ciencias sociales. Para abordar esta importante limitación, en los últimos años se ha extendido el empleo de técnicas de inferencia causal, un conjunto de procedimientos estadísticos establecidos para poder extraer conclusiones causales en investigaciones no experimentales. A pesar de su amplia popularidad y difusión en el ámbito de las ciencias sociales y de la salud, su uso en investigación educativa es todavía marginal. Así, este trabajo introduce las principales técnicas de inferencia causal disponibles para el investigador educativo cuando dispone de datos observacionales de panel. Tras abordar las características clave y el potencial de las técnicas de emparejamiento por puntuación de propensión, variables instrumentales y diseño de regresión discontinua, se presenta un ejemplo de aplicación de cada una de ellas empleando las bases de datos obtenidas en la evaluación PISA 2018. Se incluye la competencia matemática como variable dependiente en todos los modelos propuestos. Dada las diferentes características de cada una de estas técnicas, la variable independiente empleada varía en los tres modelos aplicados: asistencia a educación infantil en el emparejamiento por puntuación de propensión, expectativas académicas del estudiante en variables instrumentales y tamaño del municipio en el que se encuentra la escuela en diseño de regresión discontinua. Se concluye el artículo discutiendo el potencial de este conjunto de técnicas, teniendo en cuenta las necesidades y procedimientos metodológicos más habitualmente aplicados en la investigación educativa.
Descargas
Citas
Ali, M. S., Groenwold, R. H. H., Pestman, W. R., Belitser, S. V., Roes, K. C. B., Hoes, A. W., de Boer, A., & Klungel, O. H. (2014). Propensity score balance measures in pharmacoepidemiology: A simulation study. Pharmacoepidemiology and Drug Safety, 23(8), 802-811. https://doi.org/10.1002/pds.3574
Altman, M. (2020). A more scientific approach to applied economics: Reconstructing statistical, analytical significance, and correlation analysis. Economic Analysis and Policy, 66, 315-324. https://doi.org/10.1016/j.eap.2020.05.006
Amadon, S., Gormley, W. T., Claessens, A., Magnuson, K., Hummel-Price, D., & Romm, K. (2022). Does early childhood education help to improve high school outcomes? Results from Tulsa. Child Development, 93(4), e379-e395. https://doi.org/10.1111/cdev.13752Amini, C., & Nivorozhkin, E. (2015). The urban–rural divide in educational outcomes: Evidence from Russia. International Journal of Educational Development, 44, 118-133. https://doi.org/10.1016/j.ijedudev.2015.07.006
Angrist, J. D., Imbens, G. W., & Rubin, D. B. (1996). Identification of causal effects using instrumental variables. Journal of the American Statistical Association, 91(434), 444-455. https://doi.org/10.1080/01621459.1996.10476902
Antonakis, J., Bendahan, S., Jacquart, P., & Lalive, R. (2010). On making causal claims: A review and recommendations. The Leadership Quarterly, 21(6), 1086-1120. https://doi.org/10.1016/j.leaqua.2010.10.010
Austin, P. C. (2011). Comparing paired vs non-paired statistical methods of analyses when making inferences about absolute risk reductions in propensity-score matched samples. Statistics in Medicine, 30(11), 1292-1301. https://doi.org/10.1002/sim.4200
Barnett, W. S. (1998). Long-Term Cognitive and Academic Effects of Early Childhood Education on Children in Poverty. Preventive Medicine, 27(2), 204-207. https://doi.org/10.1006/pmed.1998.0275
Barnett, W. S., & Jung, K. (2021). Effects of New Jersey’s Abbott preschool program on children’s achievement, grade retention, and special education through tenth grade. Early Childhood Research Quarterly, 56, 248-259. https://doi.org/10.1016/j.ecresq.2021.04.001
Belitser, S. V., Martens, E. P., Pestman, W. R., Groenwold, R. H. H., de Boer, A., & Klungel, O. H. (2011). Measuring balance and model selection in propensity score methods. Pharmacoepidemiology and Drug Safety, 20(11), 1115-1129. https://doi.org/10.1002/pds.2188
Campbell, D. T., & Stanley, J. (1963). Experimental and quasi-experimental designs for research. Wadsworth Publishing.
Castro Aristizabal, G., Giménez, G., & Pérez Ximénez-De-Embún, D. (2017). Educational inequalities in latin america, PISA 2012: Causes of differences in school performance between public and private schools. Revista de Educación, 2017(376), 33-59. Scopus. https://doi.org/10.4438/1988-592X-RE-2017-376-343
Choi, A., Calero, J., & Escardíbul, J.-O. (2012). Private tutoring and academic achievement in Korea: An approach through PISA-2006. KEDI Journal of Educational Policy, 9(2), 299-322. Scopus.
Cinelli, C., Forney, A., & Pearl, J. (2022). A crash course in good and bad controls. Sociological Methods & Research, 00491241221099552. https://doi.org/10.1177/00491241221099552
Cordero, J. M., & Gil-Izquierdo, M. (2018). The effect of teaching strategies on student achievement: An analysis using TALIS-PISA-link. Journal of Policy Modeling, 40(6), 1313-1331. Scopus. https://doi.org/10.1016/j.jpolmod.2018.04.003
Courtney, J. R., Garcia, J. T., Rowberry, J., Eckberg, N., Dinces, S. M., Lobaugh, C. S., & Tolman, R. T. (2023). Measuring impact of New Mexico prekindergarten on standardized test scores and high school graduation using propensity score matching. International Journal of Child Care and Education Policy, 17(1), 9. https://doi.org/10.1186/s40723-023-00112-9
Crespo-Cebada, E., Pedraja-Chaparro, F., & Santín, D. (2014). Does school ownership matter? An unbiased efficiency comparison for regions of Spain. Journal of Productivity Analysis, 41(1), 153-172. Scopus. https://doi.org/10.1007/s11123-013-0338-y
Gamazo, A., & Martínez-Abad, F. (2020). An Exploration of Factors Linked to Academic Performance in PISA 2018 Through Data Mining Techniques. Frontiers in Psychology, 11. https://doi.org/10.3389/fpsyg.2020.575167
García-Pérez, J.I., & Hidalgo-Hidalgo, M. (2017). No student left behind? Evidence from the Programme for School Guidance in Spain. Economics of Education Review, 60, 97-111. Scopus. https://doi.org/10.1016/j.econedurev.2017.08.006
Hill, A. D., Johnson, S. G., Greco, L. M., O’Boyle, E. H., & Walter, S. L. (2021). Endogeneity: A Review and agenda for the methodology-practice divide affecting micro and macro research. Journal of Management, 47(1), 105-143. https://doi.org/10.1177/0149206320960533
Huenermund, P., Louw, B., & Rönkkö, M. (2022). The choice of control variables: How causal graphs can inform the decision. Academy of Management Proceedings, 2022(1), 15534. https://doi.org/10.5465/AMBPP.2022.294
Imai, K., Keele, L., Tingley, D., & Yamamoto, T. (2011). Unpacking the black box of causality: Learning about causal mechanisms from experimental and observational studies. American Political Science Review, 105(4), 765-789. https://doi.org/10.1017/S0003055411000414
Imbens, G. W., & Kalyanaraman, K. (2012). Optimal bandwidth choice for the regression discontinuity estimator. Review of Economic Studies, 79(3), 933-959. Scopus. https://doi.org/10.1093/restud/rdr043
Imbens, G. W., & Lemieux, T. (2008). Regression discontinuity designs: A guide to practice. Journal of Econometrics, 142(2), 615-635. Scopus. https://doi.org/10.1016/j.jeconom.2007.05.001
Imbens, G. W., & Rubin, D. B. (2015). Causal Inference for Statistics, Social, and Biomedical Sciences: An Introduction.
Jin, S. (2022). On inconsistency of the overidentification test for the model-implied instrumental variable approach. Structural Equation Modeling. Scopus. https://doi.org/10.1080/10705511.2022.2122978
Kaplan, D. (2016). Causal inference with large-scale assessments in education from a Bayesian perspective: A review and synthesis. Large-Scale Assessments in Education, 4(1). Scopus. https://doi.org/10.1186/s40536-016-0022-6
Kerlinger, F. N., & Lee, H. (1999). Foundations of behavioral research (004 ed.). Wadsworth Publishing.
Lee, D. S., & Lemieux, T. (2010). Regression discontinuity designs in economics. Journal of Economic Literature, 48(2), 281-355. Scopus. https://doi.org/10.1257/jel.48.2.281
Levi, U., Einav, M., Ziv, O., Raskind, I., & Margalit, M. (2014). Academic expectations and actual achievements: The roles of hope and effort. European Journal of Psychology of Education, 29(3), 367-386. https://doi.org/10.1007/s10212-013-0203-4
Lopez-Agudo, L. A., González-Betancor, S. M., & Marcenaro-Gutierrez, O. D. (2021). Language at home and academic performance: The case of Spain. Economic Analysis and Policy, 69, 16-33. Scopus. https://doi.org/10.1016/j.eap.2020.11.003
Maydeu-Olivares, A., Shi, D., & Fairchild, A. J. (2020). Estimating causal effects in linear regression models with observational data: The instrumental variables regression model. Psychological Methods, 25(2), 243-258. https://doi.org/10.1037/met0000226
Martínez-Abad, F., Gamazo, A., & Rodríguez-Conde, M.-J. (2020). Educational Data Mining: Identification of factors associated with school effectiveness in PISA assessment. Studies in Educational Evaluation, 66, 100875. https://doi.org/10.1016/j.stueduc.2020.100875
McCoy, D. C., Yoshikawa, H., Ziol-Guest, K. M., Duncan, G. J., Schindler, H. S., Magnuson, K., Yang, R., Koepp, A., & Shonkoff, J. P. (2017). Impacts of Early Childhood Education on Medium- and Long-Term Educational Outcomes. Educational Researcher, 46(8), 474-487. https://doi.org/10.3102/0013189X17737739
McCrary, J. (2008). Manipulation of the running variable in the regression discontinuity design: A density test. Journal of Econometrics, 142(2), 698-714. https://doi.org/10.1016/j.jeconom.2007.05.005
OECD. (2009). PISA Data Analysis Manual: SPSS, Second Edition. Organisation for Economic Co-operation and Development. https://doi.org/10.1787/19963777
OECD. (2019). PISA 2018 Assessment and Analytical Framework. OECD Publishing. https://doi.org/10.1787/b25efab8-en
Pearl, J., & Mackenzie, D. (2018). The book of why: The new science of cause and effect. Falta editorial
Pokropek, A. (2016). Introduction to instrumental variables and their application to large-scale assessment data. Large-Scale Assessments in Education, 4(1). https://doi.org/10.1186/s40536-016-0018-2
Rosenbaum, P. R., & Rubin, D. B. (1983). The central role of the propensity score in observational studies for causal effects. Biometrika, 70(1), 41-55. https://doi.org/10.2307/2335942
Rosenbaum, P. R., & Rubin, D. B. (2022). Propensity scores in the design of observational studies for causal effects. Biometrika, asac054. https://doi.org/10.1093/biomet/asac054
Rubin, D. B. (1974). Estimating causal effects of treatments in randomized and nonrandomized studies. Journal of Educational Psychology, 66, 688-701. https://doi.org/10.1037/h0037350
Rutkowski, D., & Delandshere, G. (2016). Causal inferences with large scale assessment data: Using a validity framework. Large-scale Assessments in Education, 4(1), 6. https://doi.org/10.1186/s40536-016-0019-1
Sanders, C. E., Field, T. M., & Diego, M. A. (2001). Adolescents’ academic expectations and achievement. Adolescence, 36(144), 795-802.
Song, Q., & Tan, C. Y. (2022). The association between family socioeconomic status and urban–rural and high-school attainment gaps: A logistic regression analysis of the China Family Panel Studies data. British Educational Research Journal, 48(6), 1102-1124. https://doi.org/10.1002/berj.3817
Stock, J., & Yogo, M. (2005). Testing for weak instruments in linear iv regression. En D. W. K. Andrews, Identification and Inference for Econometric Models (pp. 80-108). Cambridge University Press.
Suárez-Álvarez, J., Fernández-Alonso, R., & Muñiz, J. (2014). Self-concept, motivation, expectations, and socioeconomic level as predictors of academic performance in mathematics. Learning and Individual Differences, 30, 118-123. https://doi.org/10.1016/j.lindif.2013.10.019
Wooldridge, J. M. (2010). Econometric analysis of cross section and panel data, second edition. Revisar
Zhang, Z., Kim, H. J., Lonjon, G., & Zhu, Y. (2019). Balance diagnostics after propensity score matching. Annals of Translational Medicine, 7(1), 16. https://doi.org/10.21037/atm.2018.12.10
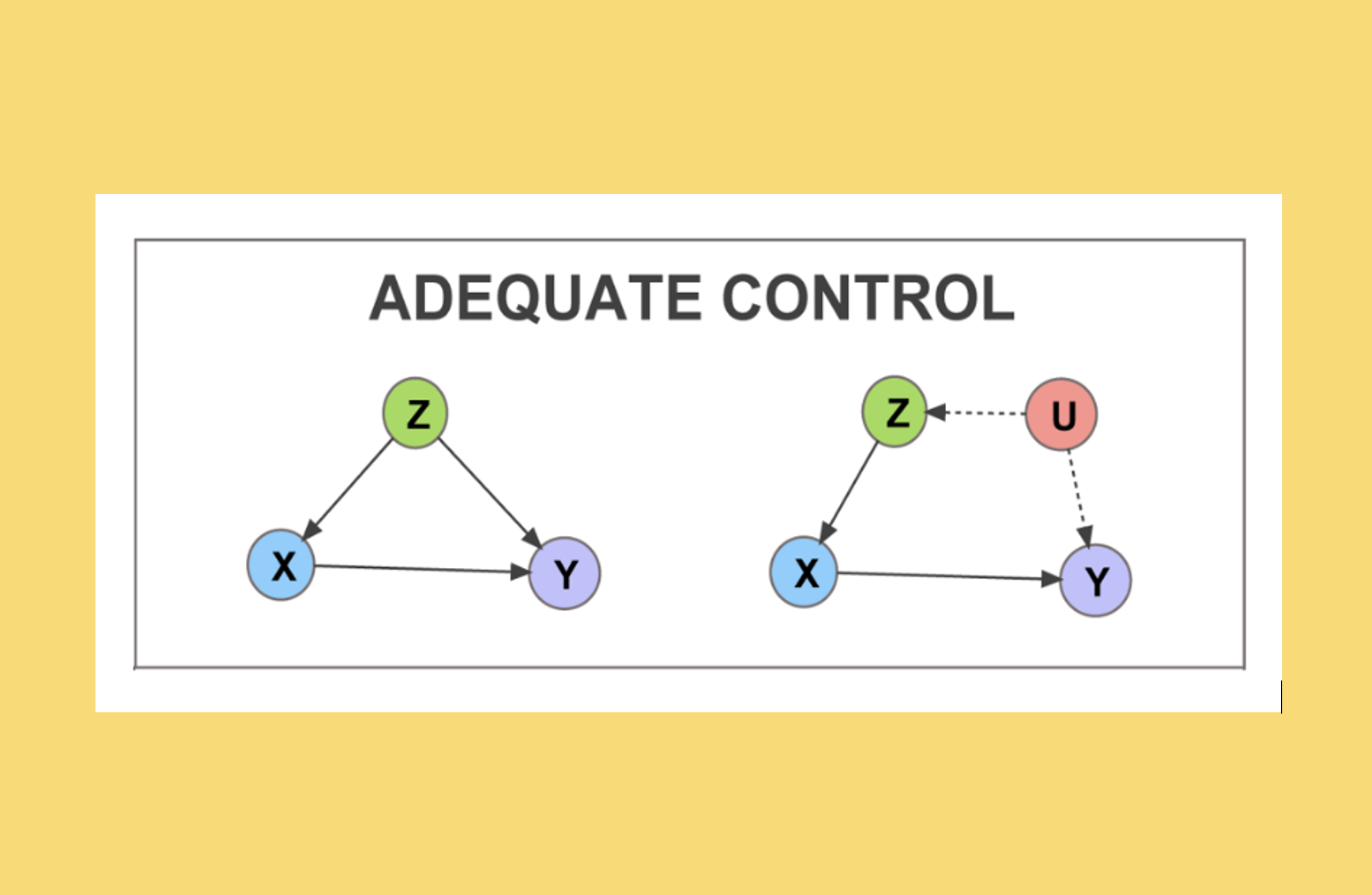
Descargas
Publicado
Cómo citar
Número
Sección
Licencia
Derechos de autor 2023 RELIEVE - Revista Electrónica de Investigación y Evaluación Educativa

Esta obra está bajo una licencia internacional Creative Commons Atribución-NoComercial 4.0.
Los autores ceden de forma no exclusiva los derechos de explotación de los trabajos publicados a RELIEVE (a los solos efectos de favorecer la difusión de los artículos publicados:firmar contratos de difusión, de integración en bases de datos, etc.) y consienten que se distribuyan bajo la licencia de Creative Commons Reconocimiento-Uso No Comercial 4.0 International (CC-BY-NC 4.0), que permite a terceros el uso de lo publicado siempre que se mencione la autoría de la obra y la fuente de publicación, y se haga uso sin fines comerciales.
Los autores pueden llegar a otros acuerdos contractuales adicionales e independientes, para la distribución no exclusiva de la versión del trabajo publicado en esta revista (por ejemplo, incluyéndolo en un repositorio institucional o publicándolo en un libro), siempre y cuando se cite claramente que la fuente original de publicación es esta revista.
La mera remisión del artículo a RELIEVE supone la aceptación de estas condiciones.